Yale University

Research
Our recent research lies at the interface of machine learning and neuroscience, and includes work on core statistical machine learning theory and methods.
The interface between ML/AI and neuroscience is a two-way street. In one direction, machine learning offers increasingly powerful tools for building predictive models that can be used to analyze neural data. More interesting and challenging is the use of AI/ML to develop complex models that can effectively act as scientific hypotheses about the brain to be tested and revised based on evidence, and evaluated against neural data. In the reverse direction, deeper understanding of cognition can feed back into computational modeling, leading to greater alignment between models of artificial and natural intelligence.
​
A recent theme in our work has been to explore models of abstraction and relational reasoning, which lie at the core of intelligence. Human cognition is driven by the ability to generalize away from low-level perceptual details and identify higher order patterns, often involving abstract categories and relations. It is important to characterize the computational and brain mechanisms that support this capacity for relational abstraction. In the past, efforts to explain this ability have drawn on two major approaches–symbolic computing and artificial neural networks–with complementary strengths and weaknesses. In contrast, the human brain achieves both in a single computational architecture.
​
With colleagues, we have been studying approaches that offer a reconciliation of the symbolic and neural network traditions, capturing the major strengths of each. These new approaches have the potential to process sensory information while also learning abstract representations directly from experience without requiring pre-specified symbols. This leads to clues about how symbol-like processing is implemented in the brain.
Much more exciting work remains to be done in this direction, both to implement these newly identified computational principles with biologically plausible mechanisms, and to use these models to form hypotheses about mechanisms of cognition that can be tested in human experiments.
​
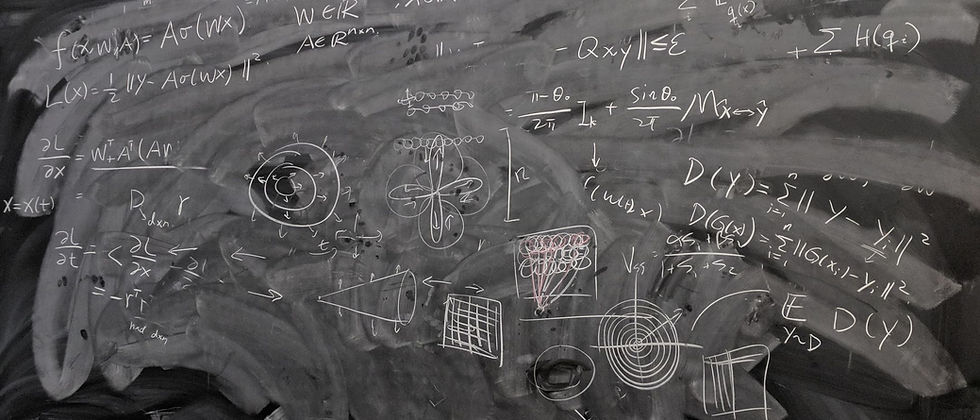