Yale University
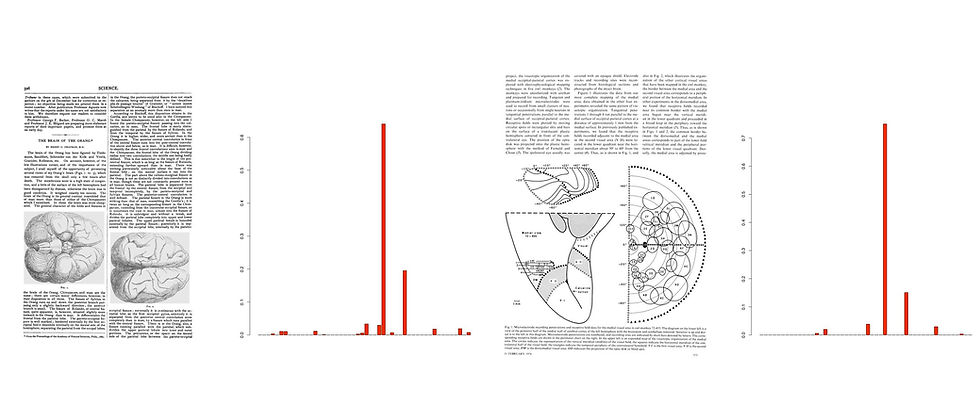
Publications
Some recent publications on a range of topics.
Relational Convolutional Networks: A framework for learning representations of hierarchical relations
Awni Altabaa and John Lafferty
​
Compositionality is essential to the success of deep representation learning.
We propose relational convolutional networks as a compositional framework for learning hierarchical relational representations.
Abstractors and relational cross-attention: An inductive bias for explicit relational reasoning in Transformers
Awni Altabaa, Taylor Webb, Jonathan Cohen, and John Lafferty
12th International Conference on Learning Representations (ICLR), Apr 2023
​
A deep architecture for relational learning is proposed that supports abstraction through a novel attention mechanism.
Emergent organization of receptive fields in networks of excitatory and inhibitory neurons
Leon Lufkin, Ashish Puri, Ganlin Song, Xinyi Zhou, John Lafferty
​
Sparse coding algorithms with local patterns of excitatory and inhibitory connections lead to receptive fields that exhibit patterns characteristic of V1, and show patterns of word semantics when applied to word embeddings in language modeling.
​
​
Shallow neural networks trained to detect collisions recover features of visual loom-sensitive neurons
Baohua Zhou, Zifan Li, Sunnie Kium, John Lafferty, Damon Clark
eLife, 2022
​Artificial neural networks with biologically informed architecture learn receptive fields that resembles thos of the compound insect eye, when trained on synthetic videos of looming objects.
​
​
Convergence and alignment of gradient descent with random backpropagation weights
Ganlin Song, Ruitu Xu, John Lafferty
NeurIPS 2021
​
We prove convergence of the feedback alignment algorithm for two-layer networks, proposed as a biologically plausible alternative to backpropgation, and show that alignment requires regularization.
​
​